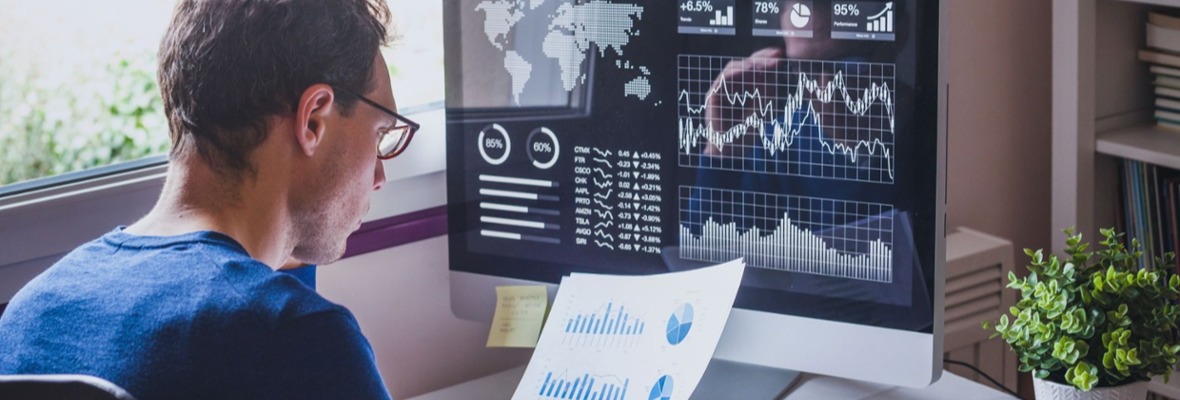
By Aaron Lyles
As the marketing analytics ecosystem continues its explosive growth thanks to the rapid adoption of marketing automation, many marketers find themselves awash in a sea of analytical tools to choose from when building their marketing analytics stack. Marketing analytics has become a non-negotiable element of any successful marketing strategy, especially in the software-as-a-service (SaaS) space.
Every marketing tool now comes with some “analytics” functionality built in, empowering marketers to track goals and key performance indicators (KPIs) across the entire customer lifecycle. A dedicated category of marketing tools has evolved just to make sense of all these disparate data sources.
This hasn’t gone unnoticed. The growing marketing analytics stack is precisely why marketers also find themselves under increasing pressure from stakeholders to prove marketing effectiveness. “Show us the money” is their mantra. After all, marketing is an investment, investments produce returns, and you have no shortage of marketing “calculators” to choose from.
However, many marketers find themselves paralyzed when asked to prove and track marketing ROI. One of the key reasons is marketing technology myopia. There are far too many tools to ever comprehensively evaluate all the options and all the ways they integrate. Where to start? One option is by conducting a MarTech audit using the MarTech Stack Audit Template from SmartBug®.
Fortunately, you don’t need to know everything. By knowing the essentials, marketers empower themselves to build sustainable, scalable strategic solutions to their marketing analytics and growth challenges. The following six-step process can help.
1. Define your marketing SMART goals
Stephen Covey’s ageless advice to “begin with the end in mind” has never been truer than in marketing analytics. Rather than becoming overwhelmed by all the possible options, it’s far better to start by defining your strategic marketing goals.
Be sure to take your time when defining your goals. At best, rushing this step will cause you to create a solution to a nonexistent problem. At worst, you’ll find yourself blindly stumbling through a never-ending rabbit hole of scope creep, with little to show for your efforts.
On that note. your goals should be SMART—specific, measurable, attainable, relevant, and timely. Again, SMART goals help protect you from scope creep that often follows vague fuzzy goals like “learning.” Learning is important and admirable, but it’s insufficient as a goal. The timeliness of your goals will also help keep your team focused, aligned, and on task.
SMART Marketing Goal Examples
- Reduce sale cycle time by 20 percent by EOY next year.
- Increase sales revenue 5 percent by Q4.
- Increase e-commerce market share by 3 percent by end of year three.
2. Determine what you need to know to achieve your goals.
Once you know your SMART goals, you can now determine what you need to know to achieve them. Your goals should keep you on track. Don’t allow yourself to be seduced by all the bells and whistles in the market.
For example, if your goal is to increase e-commerce market share, what would you need to know to achieve that goal? You might need to know how many people are visiting your website over time. It would probably help to know where they came from and which calls to action (CTAs) convert visitors into first-time customers.
It’s hard to fill a bucket with a hole in the bottom, so customer retention is likely going to be important. For example, you probably should know how often customers return to buy a second and third time. It might also be valuable to know what people say about your product to others online.
In this effort, ask yourself what’s missing in your 360-degree view of the customer. That said, stay focused on the data that you actually need to know to achieve your goals. After all, there’s unlimited data that might be interesting to know, but it won’t necessarily inform goal-oriented action.
A good place to start identifying what you need to know is to identify which KPIs will be most useful for tracking progress toward your ultimate SMART goals. Having well-defined KPIs enables strategic and operational focus for long-term marketing success. Once you know your KPIs you can identify additional necessary supporting metrics.
Common Marketing KPIs
- Marketing ROI
- Customer lifetime value (CLV or CLTV)
- Customer acquisition cost
- Click-through rate (CTR)
- Conversion rate (CR)
Common Marketing Metrics
- Marketing revenue
- Site visitors
- Email opens
- Clicks
- Bounce rate
- Conversions
- Attribution
What’s the difference between a metric and a KPI? Many marketers mistakenly use these terms interchangeably, but it’s important to recognize that they mean distinct things. While metrics are measures, KPIs are key measures that indicate how well we’re tracking against our SMART goals.
However, metrics and KPIs are not mutually exclusive. While all KPIs are metrics, not all metrics are KPIs. They exist relative to one another. It’s up to your team to determine which metrics are key. The success of your marketing analytics stack depends on getting this right.
3. Determine the source of truth for what you need to know.
Once you know what questions you need marketing data to answer, you need to determine where you will get data to answer those questions. In most cases, answers to all of your marketing questions will come from multiple different sources rather than a single definitive source. However, for any given data point, your source of truth is the single source considered to hold the definite answer.
Common Marketing Analytics Data Sources
- Site analytics (e.g., Google Analytics, Matomo, Plausible, and so forth)
- Social media analytics (e.g., Facebook, LinkedIn, and YouTube)
- Customer relationship management (CRM) analytics (e.g., HubSpot CRM and Salesforce CRM)
- Marketing automation analytics (e.g., HubSpot, Pardot, and Klaviyo)
- Ad analytics (e.g., Google Ads, Facebook Ads, and AdRoll)
It’s common during this step to identify both conflicting and missing sources. For missing sources, you need to define a data source. However, for conflicting data sources, you will need to determine which source is more reliable.
To identify a single source of truth for a data point, determine your data requirements. This includes clarifying how timely the marketing data needs to be for the specific questions it's meant to help answer. Also, you’ll need to think about data governance—how the data will be accessed, used, and controlled. The job of an interface, whether a graphical user interface (GUI) or application programming interface (API), is to assist in this effort. Keep in mind that the source of truth for a given data point may change depending on who, why, and when the data point is being accessed.
4. Determine how you will answer marketing questions and discover new insights.
Business intelligence (BI) is the ability of decision makers to monitor, explore, analyze, and process data in order to discover actionable insights. BI tools represent a rapidly growing and expansive category of applications designed to make analyzing a wide range of data types and sources more efficient and more accessible to a greater range of roles and skill levels. A few of the key capabilities of BI tools include data visualization and data modeling.
Popular Marketing BI Tools
- HubSpot
- Databox
- SAP Business Objects
- SAS Business Intelligence
- Google Data Studio
- Microsoft Power BI
- Tableau
Don’t be intimidated by platforms you don’t recognize. Marketing automation tools such as HubSpot provide more than sufficient reporting capabilities for most marketers. With HubSpot, you can even track and report attribution by channel, asset, and campaign.
By the way, taking the HubSpot Attribution Reporting Readiness Assessment is a great way to identify gaps in your marketing analytics stack.
5. Determine how marketing analytics data will be stored and managed.
With the advent of big data, data storage is an increasingly important component of the marketing analytics stack. Transactional applications such as CRM and marketing automation systems tend to suffer from data bloat.
As historical data accumulates, it often makes sense to archive older data after a certain period to keep mission-critical transactional systems running optimally. The old data can still be mined for actionable insights, but it just can’t efficiently be stored in the same place as newer data. This is where data lakes, data warehouses, and data lakehouses come into play.
Data Warehouses
Data warehouses are relational databases designed specifically for analyzing data, especially historical data such as sales history. Much like a physical warehouse, data warehouses are highly structured and well organized. Due to their relational nature, they excel at accommodating ad hoc (off-the-cuff) queries, unlike many transactional application databases (e.g., ERPs, WMs, CRMs, and so forth). One reason for this is that transactional databases must often accommodate many simultaneous read and write transactions. By contrast, data warehouses are read only.
Data warehouses tend to have two major shortcomings: their performance suffers on very large data sets, and the data they contain must be highly structured. To overcome the former issue, multiple smaller focused data warehouses, called data marts, are often used.
Data Lakes
Whereas data warehouses (and data marts) are highly structured, data lakes tend to be very unstructured and unformatted, like a pool of water. Data lakes excel at cost-efficiently storing huge amounts of disparate data from a variety of sources in unique datasets to be used at some later point which may or may not yet be known.
However, when that later utilization point comes, the analyst will first need to prepare (structure) the data before any analysis can be conducted through an extract, transform, load (ETL) process. Often, this involves loading the relevant data into a data warehouse where queries will be run.
Data Lakehouses
What if you find yourself in need of performing many ad hoc queries at scale on a very large unstructured data set? Fortunately, that need is the reason for an emerging approach called a data lakehouse. A data lakehouse is a data architecture that combines both the benefits of data warehouses and data lakes.
6. Account for the impact of machine learning and artificial intelligence.
The primary drivers of the need for both data storage speed and scale are the adoption of machine learning (ML) and artificial intelligence (AI) applications which require repeatedly querying massive data sets in various and new ways. Although ML and AI may seem very advanced or far down the road for your organization, your team should absolutely start giving these topics sufficient thought now because they require the right data architecture to be developed first.
Common ML/AI Platforms
- Google AI
- TensorFlow
- Amazon SageMaker
- Alteryx Analytics
- Microsoft Azure Machine Learning Studio
Design your marketing analytics stack for growth.
As the marketing stack continues to expand and produce more marketing data, marketing analytics becomes increasingly more important to marketing success. Companies that sufficiently grasp this concept and master marketing analytics today will be the market leaders of tomorrow. Great marketing analytics is only possible with an intentional marketing analytics stack designed to serve where a company is now and scale as it achieves its growth goals of tomorrow.
About the author
Aaron Lyles is a Sales and Marketing Strategist for SmartBug Media. He is goal-driven, profit-focused, and data-oriented. Aaron has over 18 years of sales, marketing and communications experience across a range of industries including technology, SaaS, publishing, and distribution. He has led enterprise scale data projects and prior to SmartBug he led sales and marketing for 5 years at a B2B SMB in the industrial space. Aaron holds degrees in marketing analytics and information management systems and is author of forthcoming books on sales funnel engineering and generative AI in marketing. Read more articles by Aaron Lyles.